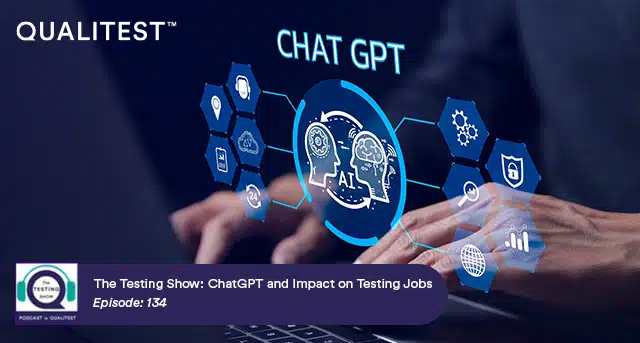
Michael Larsen (INTRO):
Hello and welcome to The Testing Show.
Episode 134.
ChatGPT and Testing Jobs.
This podcast was recorded on Thursday, March 9, 2023.
For today’s show, Beth Marshall and Chris Kenst return to continue the ChatGPT conversation, and today’s focus is on what effect, if any, Chat GPT may have on testing jobs. Is it a job killer as some fear, or is it yet another tool that will aid the smart and effective tester in being more effective?
And with that, on with the show.
Matthew Heusser (00:00):
Well we’re back one more time to talk about ChatGPT, specifically the impact of ChatGPT on jobs. To do that, we got a couple of people from our prior panel together. More of a conversational than a interview style this episode as we don’t have Michael. It’s just me and Beth Marshall and Chris Kenst. Both of them are senior members of the test community, Beth in the United Kingdom. Chris in California, last time I checked. And Beth has done a lot of really interesting things. You were doing QA relations at Mailinator, which is a tool many of us use to simulate emails, to actually get emails to go through and have captured/captive email boxes and things like that. And you’ve done a lot of other cool stuff. You were at ADA Health and now you’re at Lloyd’s Banking Group. Is that Lloyd’s of London?
Beth Marshall (00:56):
I think they’re slightly… slightly different companies. There’s lots of Lloyd’s. There’s a Lloyd’s Pharmacy too.
Matthew Heusser (01:01):
Oh wow.
Beth Marshall (01:02):
Yeah. Lloyd’s Banking Group. They’ve been around for quite a long time.
Matthew Heusser (01:05):
Like hundreds of years.
Beth Marshall (01:06):
Since the 19th century. Yeah. All guys. Yeah. <laugh>. Well I’m loving it. I’ve been there a month. Yeah, it’s fabulous. I love it.
Matthew Heusser (01:14):
So I don’t know how to describe you. How would you describe yourself.
Beth Marshall (01:18):
Beth?
Matthew Heusser (01:19):
Okay, well that’s…
Beth Marshall (01:21):
Beth’s from Leeds. That’s me.
Matthew Heusser (01:23):
Yeah, I appreciate the humility but what can our audience, I mean you’ve done a ton of testing stuff. What is your specialty within the space? It looks like fair a bit of finance, FinTech in your background.
Beth Marshall (01:35):
I’m just curious in general. But I’ve been testing for a long time and the things that I’m really passionate about is really helping to open the doors for new people to get into testing. So I love anything that can make things easier for people to have a shot at this career, in this industry, and learn more about it. Um, so anything kind of low coding, I love Postman, love API testing, all that good stuff.
Matthew Heusser (02:00):
I associate you heavily with Ministry of Tests. Is that just…
Beth Marshall (02:04):
Yeah, love Ministry of testing too.
Matthew Heusser (02:06):
And they’re at the UK headquartered sort of service-oriented test education provider.
Beth Marshall (02:14):
Absolutely.
Matthew Heusser (02:14):
What I’d say they’re a for-profit, but you can pick up service orientation in the way that they work. They’re and speaking of huge community.
Beth Marshall (02:21):
Yeah.
Matthew Heusser (02:22):
But there’s a slightly smaller community that came out of what’s called context-driven testing in the nineties I think I wanna say, which is the Association for Software Testing, which we’ve got the president of AST, Chris Kenst, who is a full-time practicing software test lead in his own right. Like he doesn’t sit at home and run association events. He does that at night. And Chris and I met at Star West in 2011 I think. Right?
Chris Kenst (02:54):
Is it Star West or STPCon?
Matthew Heusser (02:56):
It was Star West because the guy from Utah… Dwayne introduced…
Chris Kenst (03:03):
Dwayne Green.
Matthew Heusser (03:04):
I know that story because he introduced himself as Noob Tester.
Chris Kenst (03:09):
The youngYeah.
Matthew Heusser (03:09):
And I thought
Chris Kenst (03:10):
and you were confused by that
Matthew Heusser (03:11):
Young kid and I kept like condescending to him and turned out it’s just, like, his handle on Twitter. And Chris, you’ve done a lot of interesting things too. You’re at Promenade group now, have been for a while. What would you say your focus integration test? Obviously I’m looking at the resumes.
Chris Kenst (03:27):
Yeah, we’re an e-commerce company. We help small businesses. We’ve got a bunch of different smaller verticals and we help small businesses like florists build in e-commerce channels so they can compete against bigger companies. We’re helping butchers, we’re helping pet shops, we’re helping restaurants basically own their own channel instead of having to rely on like a DoorDash or a big partner that takes a big chunk of their money. We’re really about helping small businesses kind of succeed online. And I’ve been there for almost five years. I’ve done everything from being the solo tester to automation engineer to manager back down to a test lead. And yeah, we’re PHP platform and we kind of have a bunch of businesses built on that.
Matthew Heusser (04:11):
So there’s a little secret in continuous delivery. When it first came out it was mostly based on PHP and there are specific advantages for CD that we could talk about a different time. But right now we’re here to talk about not just ChatGPT, but what we all wanna know, what’s the impact of ChatGPT gonna be for jobs in the next five, 10 years or frankly, if you can see three, four months, how accurate is our crystal ball? What do you think is gonna happen?
Beth Marshall (04:46):
So I think it’s fair to say no one’s seeing any immediate impacts as of today. I’m not seeing anything rocking my boat right now. The adoption curve that we talk about is definitely at that crazy early stage where everyone is bonkers about this tool right now and it’s perhaps not reached that safe point where people are using it comfortably and it’s starting to… well I know there’s a bunch of AI tools that have been sped off the back of this thing. But I guess where I’m coming from is I’m really with… there was some research by, I think it was the World Economic Forum and they basically did a little bit of research into AI and its ilk and they sort of said the Fourth Revolution is coming and it will change things. The research they indicated said it would probably eliminate about 5% of jobs, which is a huge number. But it would augment about 50% of jobs. And I think it’s the augmentation that is the key bit of interest for testers because I don’t see it necessarily replacing testing. I see it as augmenting as becoming a increasingly critical helper to testers to help us do our jobs better.
Chris Kenst (06:03):
The augmenting. So if you look back at when new tools get introduced and its impact on a group of people, I think what happens first, AI has been around it in use in companies already and what it does now is it augments. So it’s being used on large data sets. It’s used to analyze tons of data. So instead of hiring a hundred people to go through and analyze data sets, it’s just being used by a handful of people to try and go through and understand what’s happening in data. I think there’s a lot of problems where people are trying to apply some form of AI or machine learning to these problems instead of going out and hiring a bunch of people to do things, they’re looking for AI to do that. So I think what we get initially is displacement. You think of like machines taking people’s jobs and like these mass layoffs and we’re going through, at least in the United States and kind of worldwide, we’re, we’re going through a correction in terms of layoffs. But that’s mostly companies going like, “Oops, we overhired the last two years.” It’s not them going, “This whole category of positions can now be handled by a predictable algorithm that’s 70% right, as long as you don’t ask it math questions.” And so I think what you get is a displacement. Yeah, these things are augmenting. We’re not hiring people to do this because we think we can get some machines to do it, but like we’re not gonna see layoffs, if ever, directly tied to this for a very long time.
Matthew Heusser (07:35):
There’s so much to respond to in there. So yeah, let’s start with the claim that AI is being used in businesses. I think that machine learning is being used in businesses. There are decision support systems that are being used. I read about a system analyzing MRIs. It could come up with a predictive hypothesis based on an MRI that then it would give to a human being to interpret and it would significantly cut down the amount of time that was spent and lead to a faster result. Those things are really happening. When it comes to e-commerce and retail, AI is being used to do things like look at fraud detection. Absolutely. But the way… I wanna be really careful for our audience, tell me if you disagree. The way it’s presented is like, “Oh, everybody’s doing AI.” I’ve seen a lot of these machine learning AI teams set up for traditional Fortune 500 companies you’ve heard of and they go for a year or two, they produce no results and then in a downturn like now they’re eliminated.
(08:39):
And I talked to one of the manager for one of those groups that got shut down. He spent three years. He said, “It’s really unfortunate cause we really put the groundwork in place for good AI work.” And my response was something like, “Well you were there three years. Did you help a decision maker make a better decision?” And he hadn’t. Well, that’s what happens. When your job is to help decision-makers make better decisions and they give you a million dollars a year for three years and you don’t help ’em do that. It’s possible that if anything, those initiatives are gonna have… tell me if you disagree, I’m riffing. I have no idea. But there are a lot of people whose job titles are like data scientists and I don’t know that they come up with answers that much better than a really good SQL analyst could. It’s possible that some of the things management thought they were going to get out of that eventually could be coming out of tools like ChatGPT through sending it in the data. The query interface for ChatGPT is so much more approachable than trying to write a unsupervised machine learning tool in R that it’s possible to the extent that there is displacement, one of the first people that’s hit is the data scientists.
Chris Kenst (09:57):
I think the ChatGPT as you said, the interface reduces that barrier and it gets people interested in the tech. But as you just said, then there’s companies that’ll go out and be like, “Here, let’s set a million dollar a year budget and let’s give it three years,” and then, oh, nothing turns out. I think that’s just normal businesses evaluating a tool. If we tried to apply this tool, we didn’t really have a good structure for what it was going to solve and it didn’t work out. Okay. I think that’s just what happens any time some tool gets hyped and people think there’s gonna be a problem that gets solved.
Matthew Heusser (10:32):
That’s fair. In the .com era, you would do that. You’d spend 10 million for three years to produce a website and now you can hire a software craftsman to build you one in a couple of weeks. So there’s been this compression of what it takes and I see tools like ChatGPT also further compressing it. To tie back into testing, I think there are a couple of people we know, I don’t know that I’m cleared to talk about it, but there are a couple of people who have very, very large sets of say structured test cases and very, very large sets of say functionality and very, very large sets of say bugs. If you can get those into a tool and you can get the analysis out and you can do risk management on it and you could say, “These are your buggiest, most important modules.” Now, we could always have done that before. We did it in Excel. It was really hard. So most people didn’t. But if the tool can understand those constructs, it might be able to do it for us.
Beth Marshall (11:27):
I think you make a really good point. The bar to entry being lowered and the bar to be able to do things that are now or in the past have been quite complex and needed that one person that really knows SQL or that really knows what they’re doing to get meaningful, valuable stuff out the other side, perhaps they’re the people that should be most worried because those bottlenecks with the advantage of something like ChatGPT, those bottlenecks will perhaps start to disappear. I was reading an article about a new job title. I’m convinced my six-year-old will have a job that I have no idea what it is. By the time he is in a career, it will not exist now. And one of those jobs I was reading about is something called prompt engineering, which is essentially asking something like ChatGPT the right questions, knowing how to use the algorithm to your advantage and knowing how to check what comes out. And I think that is definitely an area that perhaps might not need the skill at the higher level that someone needs to do now to get something valuable about it. So it might mean there’s more room for less experienced people to actually do something meaningful. Which personally I don’t think that’s necessarily a bad thing.
Matthew Heusser (12:47):
Well it’s like leverage. It allows you to go further to go faster to go easier. We’ve got an episode and we might do another one. Qualitest has a tool that you dump all your data into it and it does AI and ML and it was… up till now, everybody had to develop that separately. I wonder if ChatGPT is gonna help us.
Chris Kenst (13:05):
I think that’s the thing, right? If you look at the companies even in the testing space that are, “Hey, we’re gonna solve problems for you.” They’re like, we’ll solve this for everybody cuz it’s not that we understand risk based on your company, but whatever the problem is that we’re trying to solve, we understand it across these a hundred or 200 companies. There’s companies out there in the testing space that are like, “We’ve crawled every app in the app store and like we found all these bugs.” On some level that’s incredibly valuable. It doesn’t mean that you’re gonna work for me, but it lowers the barrier. It gives you a price point that you’re like, “I could try this.” So whatever it is that you’re solving now we’re talking about a few thousand dollars, whatever it is, the cost of the tool rather than, as you said, hiring somebody, you have a specialist that you’re gonna bring in, you’re gonna build this thing yourself.
Matthew Heusser (13:53):
So there’s been a lot of talk about this, I don’t know how to the extent to which you’ve worked with the tool, I’ve worked with a fair bit. In the current market environment, I see companies trying to cut costs to the extent that there’s hiring going on, it’s mostly offshore. People are trying to get that hourly rate down. I think what you’re saying is that you get to keep your job. We’ve got all this analysis data-y stuff we don’t have the time to do. We’re not gonna hire anyone to do it. Can you do that too? The job role is going to include a deeper level analysis assisted by AI.
Chris Kenst (14:27):
I think you get, you go back to this thing, generalist versus specialist. I think what ends up happening as a generalist, you end up with more tools that you can use. So instead of having to go out and need a specialist for something, no, you have a tool off the shelf that you can try and plug in somewhere to see if it works for you. To your analogy before Matt, it’s like you’re not gonna go out and spend $3 million over three years. You’re just gonna be like, “Well let’s try this tool for six months and see how well it works. Oh, we got no business value. Okay well that cost us way less money.”
Matthew Heusser (14:59):
Could it be an on-ramp to tooling and automation where you say, “Give me a Puppeteer script to go to this website, click these buttons, do these things in Python” and bam it generates you in script and you run it and it works or it doesn’t and you fix it up. And you could do that with relatively little coding knowledge or…
Chris Kenst (15:21):
It’s the thing you try right now in ChatGPT is one of the ways you test it is you go, “Give me a Python script” and you can go, “All right, well that looks totally wrong” or “That looks reasonably complete.” Yeah.
Matthew Heusser (15:35):
I did some playing, I don’t know if this is talk about this in the last show, but I did a fair bit of playing with tool to try to generate code and it got better, specifically Selenium. I think there were other programmers training it cuz it learned from everybody. And what does Selenium even mean? It might give you an example for Selenium IDE cuz it just crawls, but it seems to pick up context and it seems to have been trained and the first time I asked for a tool to do a Google search for certain term and click on the result and do this, it produced it. And that Python script which I generated through AI is out there in GitHub and I don’t think I own it, right? You’re not supposed to be able to copyright the results in the tool, but I think it’s been trained.
Beth Marshall (16:21):
I think the brilliant experiment that I would love to see is since we recorded the earlier podcast and this one, GPT4 has been released.
Matthew Heusser (16:32):
Right.
Beth Marshall (16:32):
Which is meant to be a little more improved and more realistic, I would love to see the results of a tester’s GPT3 versus GPT4 and see if that code is any better and any smarter. That’d be interesting. I did try and log on, but the net has started to close on people that want to use this tool for nothing and I think they are restricting users that aren’t in their premium model. So that’s happened quite quickly. <laugh>. They’ve built up their fan base so quickly that they’ve been able to draw that, draw a bridge up.
Chris Kenst (17:07):
Says Beth, the tutorial writer on Postman. So what you could probably do is you could probably hit their API and select the old models versus the new models and run that same prompt through. Right? I think we just found Beth’s new video post.
Beth Marshall (17:23):
My new hobby, <laugh> new hobby.
Matthew Heusser (17:25):
And I think it’s, I think it’s $50 a month. I was reading that a Google search costs 5 cents, three to 5 cents. That’s for the infrastructure, for the web crawlers and maybe for the employees, I don’t know. So they have to capture 6 cents in revenue for every Google search. ChatGPT, I’m sure, GPT4 is going to require more processors, require 30 cents for one set of search results. So they gotta find a way to monetize that the way they’re doing. It’s through paid memberships for now.
Chris Kenst (17:56):
So the other thing Beth mentioned, GPT4 is out and then I think a week or two prior, they also announced that they had modified their models and they decrease pricing by like 50 or 90% or something. They went from, I forget what it was priced at and I forget how they do their billing, but it’s like tokens. They went down to like 3 cents. So it was, they cut the price massively for the last few iterations of their models. But that doesn’t mean that they’re profitable, that they’re making money. But the point being that they are decreasing the price rather than increasing the price. And they have seen, as Beth said, just an increase in the amount of users.
Matthew Heusser (18:41):
That’s impressive.
Beth Marshall (18:42):
In my entire lifetime. I’ve never known a piece of technology that has become so ubiquitous so quickly. It was on the news last night, the prime ministers, oh there’s someone over here in the UK. The chancellor was getting it to write his speech and it’s everywhere and it feels like it’s happened overnight. And I think that’s one of the cornerstones of this Fourth Revolution that we’re going through that things just will happen a lot faster. Yeah, I think that’s something we all need to do. We all need to get used to it or should we be a little Luddite about it and try and resist and have a healthy criticism because there… it is not perfect at all, right? There’s huge detractors from this thing.
Matthew Heusser (19:28):
Yeah, if you ask, it’s something that is not, if it’s not easily able to put it into a symbolic representation and then do a logical transformation on it, if you’re not asking it to solve math problems, then it’ll go through the whole internet, which as we know is “completely reliable”. And we’ll then sort of cut and paste paragraphs together from different sources and then try to rewrite them so that they’re not legally the property of the place that it’s sourced from and the result can be a mess. So ubiquitous is interesting, like you hear about it everywhere. “Oh, this is the hot new thing.” But I don’t know the extent that it’s really being used, like the actual level of adoption and there’s, it’s not the diffusion of innovation curve. There’s another one, is it McKinsey has a curve for technology innovation and that one it’s a hype cycle.
(20:23):
Yeah, the McKinsey’s hype cycle, you start with the peak of inflated expectations, which is where we are. And then we go through the trough of disillusionment and then eventually figured out what’s actually good for, and you have the plateau of productivity and I think we’re way up here cuz like really we don’t know. We don’t have a lot of concrete examples and we really try to keep it grounded in practical experience here. We just keep hearing about. So I got an idea for you when we came out with cell phone with geolocation, we’re gonna know where you are. I read this article, I don’t know what, 10, 15 years ago it said that everything’s gonna be connected on the internet of things. Your soda machines are gonna be on the internet of things and they’re gonna know the weather. And then when it gets hot and it’s high humidity or low humidity, then to increase the price of the soda cuz people will buy it anyway.
(21:14):
And when you walk by a soda machine on your phone, it’s gonna be geo aware, it’s gonna pop up, text you a coupon to get that soda on sale, right? None of that stuff happened. But we did get things like retargeting. We did get things like, you can put a geo-fence around your retail establishment and when people are driving by you can say, “I want to send ads to people that are driven by my store lately that also bought a book from Borders”, whatever. And all of that infrastructure now works, which is a better extrapolation from the idea that I mentioned earlier with the soda machine. So what I’m trying to say here is I would guess that most of the examples that people come up with are not very good, but what the actual application of the tool is we’re gonna have to see. And that also goes into how it’s gonna impact our work on a day-to-day basis. I would like to hear Beth talk a little bit about how she sees low-code, no-code tools impacting because I think that’s a lot closer to real.
Beth Marshall (22:19):
Yeah.
Matthew Heusser (22:19):
Still hasn’t had much of an impact.
Beth Marshall (22:21):
I think that all plays into making this stuff easier and easier. So there’s a reason why I don’t know binary. And that is because I don’t need to use it. And I kind of think that the more abstraction layers we will have, the easier it becomes for people to not be scared by technology. There’s still a huge amount of the population that have great ideas but have no idea how to implement it. There’s also a huge amount of smoke and mirrors in technology as well that people put the guardrails down and don’t let other people in. And we are missing out on a huge amount of talent there that’s just scared off by tech. And I think that’s a shame. The other thing that I think ChatGPT can help with, to go off on a slight gender tangent for a moment, I wrote a blog recently about kind of women’s work and how a tool like ChatGPT can maybe help with some of the glue work that often women are asked to do.
(23:24):
For example in tech, “Oh, can you just review this document for me? Everyone else reviews it and they just say it’s fine, but you are really good. You are really thorough.” Maybe ChatGPT could just do that for you and you don’t need to tell anybody.
Matthew Heusser (23:38):
Oh, I see what you’re saying.
Beth Marshall (23:39):
I think there’s perhaps little instances like that where there is hidden work that is often unrewarded, undervalued, but essential to keep the wheels greased on teams. And I think ChatGPT could maybe be a little win there.
Matthew Heusser (23:56):
Yeah.
Beth Marshall (23:56):
Because it does fall predominantly on the shoulders of women, statistically.
Chris Kenst (24:00):
Well, and then did you just describe testing work by saying undervalued? Not always recognized?
Beth Marshall (24:06):
Maybe you’re right.
Chris Kenst (24:07):
Maybe.
Beth Marshall (24:08):
What do you think?
Chris Kenst (24:09):
Yeah.
Matthew Heusser (24:10):
But at least with the tester role, it’s official, it’s the job. What Beth is talking about is work that it’s not even recognized. Or worse, the person who wrote the article and got the copy editing or whatever it was for free gets all of the credit. We recognize that work is done and we attribute it to the wrong person. So I think that that also happens to people that are slightly extroverted and highly agreeable. And I have a highly agreeable personality. So that same thing happens to me. You look back and how many times was I the secretary of the nonprofit cuz somebody had to do it, maybe Matt can do it. And I kind of leaned into it personally, but I found ways to get credit for it. So I think that’s the danger and I totally hear what you’re saying. That makes a lot of sense to me.
(24:54):
Fair enough. I think it could have an impact. I’m not sure what most organizations are tightening up their budgets, but not all. And it could be another resource or thing to pull. And I’ve said this before, I think it’s true. I think ChatGPT is a pretty good college freshman research assistant. In terms of the hierarchy, as with the Dreyfuss Model of Knowledge? First you just have sort of memorization. Like I can ask a question, split out the answer. The 100 level in college, the freshman level in college, it’s memorize all the parts of the body, memorize all the different kinds of animals, and you get to the higher levels, you get to comparison, application and creation. I think ChatGPT is pretty good at that. You know, summarize this Supreme Court result and it could blitz out an answer. It’s actually a summary of all of the other summaries. It would give me the first place to start when I actually do my own work. So what does that look like in testing? You mentioned reviewing design documents, reviewing requirements.
Chris Kenst (25:52):
Yeah, reporting is a big deal in testing. Summarizing the work that you did is very important and being able to make it actionable and useful for your stakeholders. That reminded me. So I use Notion, which is just a tool for everything. Like you could put just notes and that kind of stuff. They, I think are using, not ChatGPT, but they’re using OpenAI’s APIs to embed that. And you can take a whole document full of information and say, summarize it for me and it’ll go through and summarize it. Putting that back, if you have test results, if you have things that you’re trying to do now, it wouldn’t do what I wanted it to do, which was actually loop through a table and analyze the data for me. Tell me some interesting patterns that I might have missed. It wouldn’t do that. So it’s very limited. But yeah, I think reporting is a big deal. And so if you’ve done a lot of work and you can find some way to summarize that data quickly. There’s a question of accuracy, but I think that’s really beneficial.
Beth Marshall (26:49):
There’s a question of trust too, right? So if you are, I think if you openly acknowledging that you are using this tool to, for example, come up with test case ideas or generate test data, I think you will struggle to get the whole support of the rest of the people in the organization for relying on that without any kind of human eyeballs on it.
Matthew Heusser (27:13):
Well, yeah, it’s very analogous to when there’s this push, get continuous delivery to not have a human check. I want a human check until we’ve delivered it consistently, however many times in a row, a hundred times in a row. And then maybe we don’t need that. But if we’re finding problems along the way, you gotta keep checking it. And at this point with the AI tools, that’s where we’re at. With the human speech, generative text tools.
Beth Marshall (27:38):
Do you think we will almost have a bit of blind faith in this technology until something goes desperately wrong and then we’ll need to rope it back? In a very broad sense, not in a particular company sense. A lot of money was wiped off the stock of a ChatGPT alternative, wasn’t it? Because… I think it was Google’s. Yeah, because in their advertising, the generated things that they were talking about were incorrect, were factually incorrect. And a human said, what does this model come up with? Hundred billion dollars, gone. Have people learned the lesson from that now? Or do you think we’ll see more of that before people become a little cautious?
Chris Kenst (28:18):
The question of “Will people learn from failures that other people have?” I think it’s debatable. Do we learn from the failures? I don’t think so because especially if you can convince yourself that, “No, that wasn’t it. What does Google know?” Right? That was a PR blunder. No, listen, I think you’re right. If there’s a hype cycle, I think people get really interested in it. They’ll try it, they’ll figure out if it doesn’t work, they’ll abandon it. They’ll be people that continue to work on it because they see value on it. I don’t think we learn lessons from this stuff. And I think part of that, it just takes a very long time to learn those lessons. It’s been 20 plus years since we’ve had Facebook and we’re still learning about all the downsides there are to social media and connecting. There’s a whole lot of research that has to connect with it. And I know that AI’s people have put forward these ethic panels and groups to try and have safety around that kind of stuff, but those are the first ones that get laid off. And so it takes time for any of this stuff to have been out there before we start to really unravel the box that we opened. And that goes with jobs, with safety, with everything.
Matthew Heusser (29:26):
Yeah, I think there’s two extremes and the one extreme is this is junk, it doesn’t work, it’s terrible, throw it away. And the other one is it’s gonna solve all our problems. And I see us as a society bouncing between those two extremes with almost no concept of there should be some middle ground for the next six to 12 months. I don’t think any big decisions that are gonna impact testing jobs that aren’t already on the way are going to happen because of this over the next six to 12 months. And I will say this, testers are a self-critical community. We like to be the person sitting in the back that says, this is never gonna work. We love to do that. We’re good at it. When I was on the board of the Association for Software Testing, we had seven of the best known, if not the best, software testers and good luck getting any idea ever approved because we were all really good at shooting down ideas.
(30:22):
That’s what we were, we’re good at that. That’ll never work. Like it was a miracle. People that criticize AST, I’m like, “No, it’s a miracle we get anything.” I don’t mean that rudely, but that’s the tester orientation is to find the risks, to find the problems and then to pick at them. This is a technology that I hope we actually give a shot at. Michael Kelly, out of Indianapolis once told me they had this opportunity to do performance testing and no one wanted to do it. It was, as you said earlier, unappreciated work. “Can you do this thing in your free time?” Which nobody had any. And if you did it and you succeeded, someone else would take credit and if you failed you’d be in trouble. No one would do it. And they were all secretly a little intimidated. And Mike Kelly was able to say, Well, I’m just the”… his word’s not mine.
(31:06):
“I’m just the dumb tester here. I’ll give it a shot. And if I can’t succeed, well I’m just the software, I don’t know anything. Anything.” That’s how he got performance testing on his team. We could do something similar with this AI tools and then we’re the ones championing them, we’re the ones driving them forward. We’re the experts and that’ll change the conversation, I think, about the role of test and quality in delivery because in too many companies and too many teams testing is you do the stuff someone who never thought about it tells you to do and then it’s low value. And then why isn’t QA more effective? I’m interested in any technology that can help us go the other direction and we should probably do closing thoughts. So thank you both for being here. I thought this was great. Anything you wanna add before we get going?
Chris Kenst (31:55):
I think the whole point of this is that there are some things that we can see, and as Matt said, Tess is really good at picking apart things. And I think that’s what we have to continue to do with ChatGPT, with AI, is just continually pick these things apart but also look to see where there’s value in how we can use it. The question of whether or not AI and to what degree impacts displaces causes problems with employment. I think that’s so far off. We should keep an eye on it and we should try and pick apart the problems and highlight them when we can, just like we do as testers. But I think that’s the extent to where we’re at. We just need to keep learning more and keep pressing on because I think these tools are incredibly valuable. We just have to figure out where.
Matthew Heusser (32:40):
Thanks, Boss. Beth? I’ll let you have the last word.
Beth Marshall (32:43):
Ooh, last word. Okay. I would just encourage people if you are unsure about this tool, if you are unsure about tech in general, by all means make sure you’re aware of its deficiencies, but play with it. Have an open mind, do some research, see what amazing things other people are doing in this space and use it to your advantage because there are things we can do right now as testers, whether that’s overtly or covertly, to give us a leg up with this. And I think especially in times as we were talking about where not every job is perhaps as secure as it has been, having those advantages can be really helpful on a personal level. So I would just try and encourage people to embrace it critically. <laugh>.
Matthew Heusser (33:34):
Well thanks, Beth. Thanks everyone, and we’ll hope to have you again on the show real soon. Thank you.
Beth Marshall (33:39):
Thanks for having me. Bye.
Chris Kenst (33:40):
Thanks. Bye.
Michael Larsen (OUTRO):
That concludes this episode of The Testing Show. We also want to encourage you, our listeners, to give us a rating and a review on Apple podcasts, Google Podcasts, and we are also available on Spotify. Those ratings and reviews, as well as word of mouth and sharing, help raise the visibility of the show and let more people find us. Also, we want to invite you to come join us on The Testing Show Slack channel, as a way to communicate about the show. Talk to us about what you like and what you’d like to hear, and also to help us shape future shows. Please email us at thetestingshow (at) qualitestgroup (dot) com and we will send you an invite to join group. The Testing Show is produced and edited by Michael Larsen, moderated by Matt Heusser, with frequent contributions from our many featured guests who bring the topics and expertise to make the show happen. Additionally, if you have questions you’d like to see addressed on The Testing Show, or if you would like to be a guest on the podcast, please email us at thetestingshow (at) qualitestgroup (dot) com.